SARS-CoV-2 or COVID-19 has dramatically affected the world in way that hasn’t been seen in over 100 years. Everyone in the world is currently feeling it’s impact and the scientific community is working around the clock to find answers. Despite our efforts, we are still without a vaccine or reliable treatment for this disease.
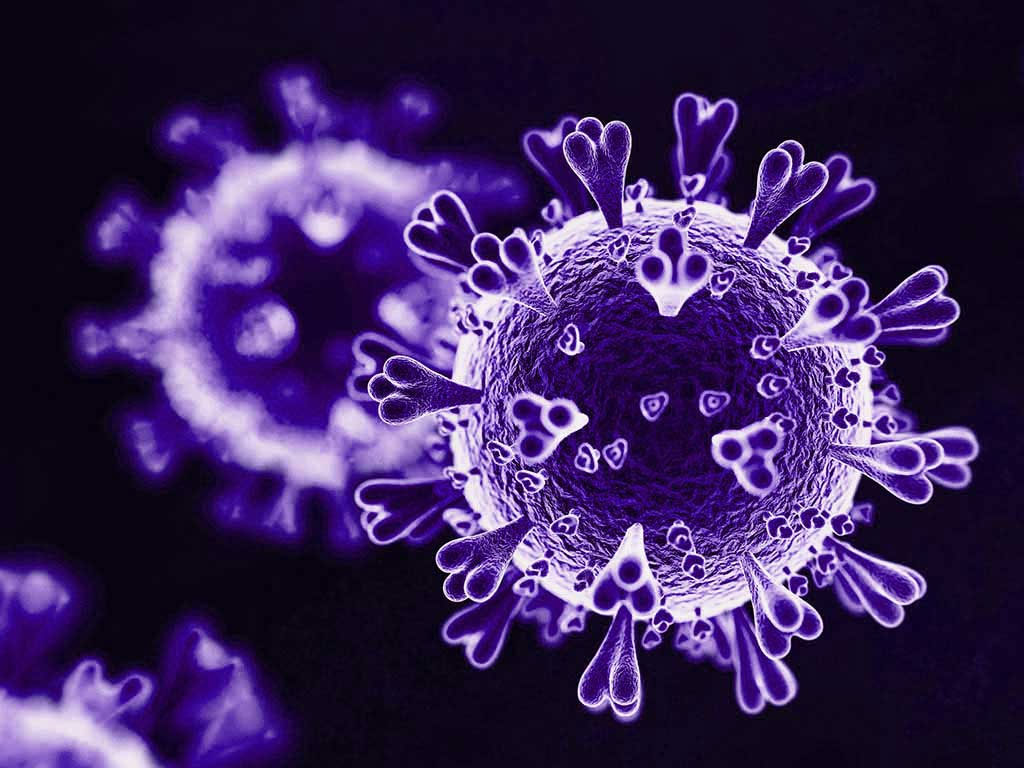
Working to find possible treatments, researchers at Recursion along with colleagues at Utah State University used high throughput screening for rapid identification of potential treatments for COVID-19. They infected normal human renal cortical epithelial cells (HRCE), normal bronchial epithelial cells (NHBE), and Caco-2 cells with SARS-CoV-2. Vero cells were also infected as a control. Cells were then analyzed using a phenotypical software analysis tool that the researchers refer to as a “deep learning-enabled drug discovery platform.”
A number of drugs and compounds were evaluated using their software platform that provides rapid image analysis to quickly determine potential treatments. The phenotypic evaluation is accomplished by an algorithm that can analyze cytological structures of cells with poor morphology thus enabling quick assessment of the efficacy of treatments.
The only antiviral in their assay that showed efficacy is remdesivir. None of the other antiviral drugs tested including chloroquine and hydroxychloroquine showed any benefit in the human models that were screened. The researchers did observe some small benefit to the cells using beta-blockers, mTOR/PI3K inhibitors and Vitamin D equivalents. There was some increase of the viral phenotype using a beta-agonist.
As we start to cautiously reopen our world again, hopefully this type of approach can narrow the number of potential candidates for treatment and contribute to faster recovery of patients.
For more information, please check out the full article for rapid identification of potential treatments for COVID-19.
Please note that the research article described in this blog is currently a preprint, which means it has yet to be peer reviewed.
Written by Joseph
Scientific Support Specialist, Lonza Pharma-Bioscience Solutions at Lonza